IoT Analytics: Reaping Value from IoT Data
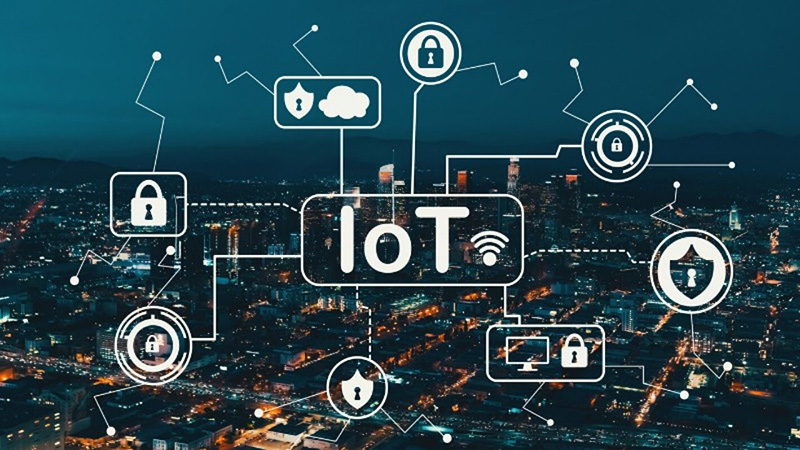
The Internet of Things (IoT) is increasingly becoming a key component of many companies’ data-driven transformation strategies. Organizations that have embraced IoT are already seeing benefits such as improved operational processes, better inventory management, and enhanced equipment maintenance — to name a few.
However, a successful IoT strategy involves more than just connecting a bunch of devices and sensors to the internet and gathering data from these “things.” IT must establish the ability to effectively analyze the vast amounts of data IoT creates to make sense of it and gain real business insights.
That’s why an analytics strategy for IoT should be a top priority for any company looking to maximize the value of all the connectivity.
Organizations can enjoy numerous advantages by leveraging the IoT data they gather, says Carlton Sapp, Senior Director and Research and Advisory Leader at Gartner.
These include contextual awareness of equipment and systems; improved decision-making, optimization, and supervisory control of equipment and resources; reduced costs associated with data management; proactive, predictive, and prescriptive management of equipment; and environmental compliance.
These opportunities are pervasive in use cases such as fleet optimization and management, asset management, financial risk management, and smart cities, Sapp says.
However, they require a sound, streamlined approach to the data end of IoT. Here are several tips for dealing with IoT data and getting the most out of these resources.
Build an IoT Analytics Organization and Infrastructure
Once an organization has an idea of its IoT analytics business goals, it needs to identify the key stakeholders who will be involved, says Stacy Crook, Research Director for IoT at IDC, and ascertain whether those stakeholders require additional skills to make the project successful.
“It is a well-known fact that data science skills are in short supply in the industry, but these are essential for IoT analytics projects,” Crook says. “So the project may require hiring new employees or outsourcing certain parts of the project to third parties if in-house data science skills are thin.”
Organizations should also consider appointing a Chief Data Officer (CDO) to champion IoT data analytics efforts and lead the data governance strategy, Crook says.
Because IoT is essentially a big data problem, IDC suggests organizations consider how their existing infrastructure could also serve IoT use cases. “Although older big data architectures might have been focused on batch-oriented workloads, increasingly there are tools available to run real-time workloads over this same backbone,” Crook says.
Leveraging the same infrastructure for various IoT workloads can have benefits in terms of preventing data silos and providing the ability to more easily run cross-functional data analysis across those workloads, Crook says. “It can also provide data governance and security benefits,” she adds.
Deploy an Architecture that Supports IoT Data Growth
Companies need to start with the right IoT data architecture and understand how to manage IoT data at various locations.
“Data emanating from IoT endpoints presents new and unique challenges, such as unreliable network access and the need to combine devices that may be distributed over large distances, generating data in multiple formats and using multiple protocols,” Sapp says.
Today, most IoT data is telemetry data, but endpoints are increasingly emitting image and audio data that should be handled by persistent data stores, Sapp notes. “Start with an appropriate IoT data architecture that will support the expected growth in the volume of IoT data,” he advises.
Organizations often fail to effectively manage IoT data due to a lack of a flexible and elastic data architecture. “Data will continue to grow, so design an architecture that leverages analytics and data mining techniques to identify critical information that can be used to improve processes, enhance decision-making, or reduce costs,” Sapp says.
For example, telecommunications companies successfully reduce the cost of moving data over a network by leveraging IoT analytics at the network edge, which helps reduce “noisy data.”
“Those organizations focus on scalable, edge-centric data architectures that are designed for rapid knowledge discovery in IoT data,” Sapp adds.
Deliver Analytics Across Data Pipelines
The IoT data architecture should also support analytics across data pipelines (via streaming) and in local data stores to leverage faster decision-making and reduced costs, Sapp says.
Organizations can achieve this by focusing on data-centric design patterns when creating and deploying IoT analytics, including the use of event-driven architectures.
“Start by distributing analytics at the edge, on streaming pipelines, on the platform, and in the enterprise,” Sapp says. Organizations should utilize streaming IoT data pipelines as a source to deploy analytics, improving latency, reducing costs, and minimizing security vulnerabilities, he adds.
For example, the U.S. Department of Defense often performs analytics over streaming data pipelines to reduce the throughput of data over a network, Sapp says. It also leverages IoT edge analytics to avoid sending data over the network, using operational analytics closer to the data source.
There will likely be multiple analytical environments deployed to support various analytics needs, Sapp says. “Environments may range from operating systems to embedded analytics software,” he notes. “Be prepared to deploy IoT analytics across a landscape that extends from the network edge to the corporate enterprise. For example, utility organizations distribute IoT analytics across various infrastructures to support fleet management.”
Leverage Artificial Intelligence
Organizations should enhance their capabilities with IoT data by leveraging AI, Sapp says.
“Edge intelligence is an emerging field that uses AI as an analytic method deployed at the network edge to develop intelligent applications from IoT data,” Sapp explains.
These intelligent applications range from video surveillance to intelligent supervisory control and data acquisition (SCADA) systems. For example, environmental organizations use IoT data to build intelligent control systems to maintain environmental compliance.
Adding AI to the IoT architecture is becoming an operational imperative, Sapp notes. IoT systems, including endpoint devices, must become smarter and more autonomous to handle the ever-increasing magnitude of data. To make these systems smarter, organizations need to deploy AI and machine learning.
Be Cloud Native
Given the massive volumes of data generated by IoT applications, the cloud is often the only solution for effective data management and analytics.
“It’s not practical to build the scale and speed needed to manage this volume in real time on your own,” says Greg Meyers, Group CIO and Chief Digital Officer at Syngenta, a company that produces agrochemicals and seeds.
“Trying to manage it yourself in your own data center or on your own infrastructure is highly self-defeating,” Meyers adds.
IoT provides Syngenta with the ability to manage its customers’ farms and fields, which are usually divided into small micro-segments. “Humans are great at managing averages, but computers are better at managing variability,” Meyers says. “IoT allows us to understand why things happening in one area differ from things happening just 100 meters away.”
Leading public cloud vendors offer services to assist companies with IoT analytics. For example, Amazon Web Services (AWS) provides IoT Analytics, a managed service that enables companies to run and operationalize sophisticated analytics on massive volumes of IoT data without the cost and complexity typically associated with building an IoT analytics platform.
Microsoft offers Azure IoT, which includes a data analytics service called Azure IoT Central, providing capabilities to examine historical trends and correlate various telemetries from connected devices. Google provides Cloud IoT, a set of tools to connect, process, store, and analyze data both at the network edge and in the cloud.
Prioritize Data Governance, Security, and Privacy
Organizations need to ensure they have governance, security, and privacy mechanisms in place for IoT data analytics processes. Much of the data generated by IoT will be sensitive or have competitive value and needs to be carefully managed and protected.
“Reassess current data governance practices to include machine data,” says Nicholas Colisto, Vice President and CIO at Avery Dennison, a manufacturer and distributor of adhesive materials, apparel branding labels, and tags.
“From my experience, IoT governance is an immature area,” Colisto notes. “In a previous company, I faced a situation where a business unit deployed an IoT system without seeking IT involvement, and simple operational tasks and tools for auditing devices and applying firmware were not considered.”
Companies need to evaluate IoT data risks based on confidentiality, privacy, and retention requirements, Colisto advises. “For example, if you are working with personal data, consider the issues that can arise from algorithmic bias or the inability to comply with regulations such as GDPR [General Data Protection Regulation], which can lead to legal action and damage your company’s reputation,” he says.
Leverage IoT Data for New Revenue Opportunities
Data generated from IoT can be valuable both inside and outside the company.
Chemical manufacturing company Texmark Chemicals launched an effort to modernize operations at its plant by deploying sensor-enabled pumps. Using technology from Hewlett Packard Enterprise and Aruba Networks, the company gathers operational data from pump sensors that measure temperature, pressure, vibrations, flow, and power. This data is analyzed to predict equipment failures before they happen.
Through a “workshopping” process, Texmark realized that having sensor-enabled equipment not only helps the company monitor its assets and processes but has also opened the possibility for new business models, says Doug Smith, CEO.
The use of IoT becomes an additional selling factor before contract negotiations, Smith notes. “Clients are beginning to realize the value of having access to data coming from contractor assets,” such as industrial pumps, he says. Clients then ask Texmark to add sensors to their pumps and provide them with the data.
“In essence, we are developing a library of historical performance attributes that can be cataloged and shared with other companies using similar equipment,” Smith says. “When deploying machine learning models, the more data acquired, the greater the accuracy of the analytical predictions.”
By sharing IoT data with pump manufacturers or fellow suppliers, “we could validate the new business model, as long as the documentation is clear and precise,” Smith notes. “Meanwhile, customers are impressed that we have deployed instrumentation and software analytics to capture, analyze, and report on such data — allowing for more cost-effective decisions.”
This new data-as-a-service offering enabled by IoT can distinguish Texmark from competitors, Smith adds, and creates a stronger bond with customers while empowering employees to achieve more from their work.