Unlock Insights Through AI-driven Analytics
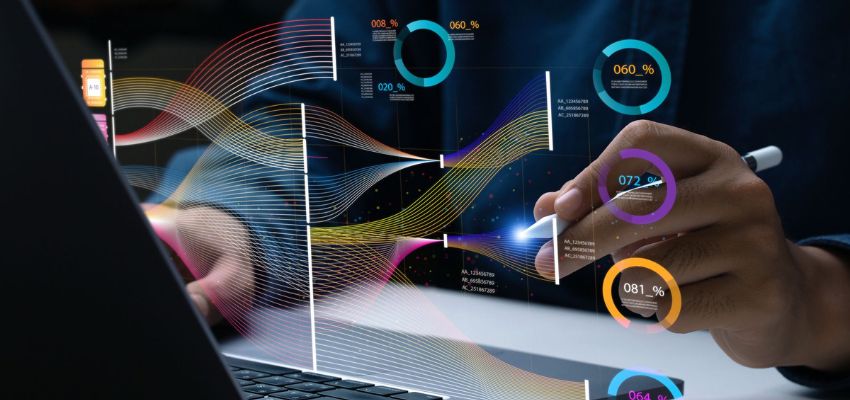
In today’s rapidly evolving technological landscape, the term AI-driven analytics has emerged as a beacon of transformative potential in various industries. This innovative approach leverages artificial intelligence to process and analyze vast amounts of data, offering businesses insights that can significantly enhance decision-making, increase efficiency, and foster growth. With its ability to uncover patterns and trends that would otherwise remain hidden, AI-driven analytics is revolutionizing the way organizations operate, enabling them to stay competitive in an increasingly complex marketplace.
Understanding AI-driven Analytics
To harness the power of AI-driven analytics, one must first grasp its core principles and components. This section will delve into what constitutes AI-driven analytics, its key features, and its overarching impact on various sectors.
Defining AI-driven Analytics
AI-driven analytics is a methodology that combines advanced statistical analysis with machine learning algorithms to derive insights from data. Unlike traditional analytics, which often relies on historical data reviews and human intuition, AI-driven analytics automates the data exploration process.
This automation not only speeds up the analytical process but also enhances accuracy by minimizing human error. The integration of AI allows for real-time analysis, which is crucial for making timely business decisions. By continuously learning from new data inputs, AI systems can refine their predictions and recommendations, leading to more informed strategies.
Understanding this definition highlights that at its core, AI-driven analytics is about leveraging sophisticated technology to turn raw data into actionable insights. It empowers organizations to ask complex questions and receive intelligent answers based on comprehensive data evaluations.
Key Features of AI-driven Analytics
Several defining characteristics set AI-driven analytics apart from conventional analytical methods.
First and foremost, it offers predictive analytics capabilities. Organizations no longer have to rely solely on past performance metrics; they can forecast future trends based on current data patterns. This dynamic forecasting helps businesses to strategize proactively rather than reactively.
Another significant feature is natural language processing (NLP). AI-driven analytics tools can analyze unstructured data, such as customer feedback and social media interactions, converting qualitative information into quantifiable insights. This ability broadens the scope of analytics beyond mere numerical data, allowing organizations to understand the nuanced sentiments of their customers.
Moreover, AI-driven analytics incorporates visualization techniques that simplify complex data interpretations. Visual representations can help stakeholders quickly comprehend insights without diving deep into extensive reports.
Ultimately, these features allow businesses to enjoy a holistic view of their operations, paving the way for improved decision-making frameworks.
Impact on Various Sectors: A Deep Dive
The implications of AI-driven analytics extend across numerous industries including finance, healthcare, retail, and manufacturing.
In finance, for instance, AI-driven analytics enhances fraud detection mechanisms. Traditional monitoring systems may struggle to identify irregular transactions until after they have occurred. However, by utilizing machine learning algorithms, financial institutions can detect anomalies in real-time, mitigating risks before they escalate.
In the realm of healthcare, AI-driven analytics assists in patient diagnosis and care management. By analyzing patient histories and treatment outcomes, AI systems can recommend personalized treatment plans, ultimately improving patient outcomes and optimizing resource allocation.
Retailers have also begun to capitalize on AI-driven analytics to optimize inventory management and personalize marketing efforts. By understanding purchasing behaviors through data analysis, companies can tailor offerings to individual preferences, thereby enhancing customer satisfaction and loyalty.
Finally, manufacturing processes benefit from AI-driven analytics through predictive maintenance. Machines equipped with sensors generate data that AI analyzes to predict when equipment is likely to fail, allowing for timely maintenance and minimizing downtime.
As can be observed, the influence of AI-driven analytics is pervasive, providing substantial benefits to organizations regardless of their industry focus.
The Role of Machine Learning in AI-driven Analytics
Machine learning stands at the forefront of advancements in AI-driven analytics. This section explores how machine learning enhances the capabilities of AI-driven analytics and contributes to the generation of valuable insights.
Understanding Machine Learning
Machine learning is a subset of artificial intelligence that empowers systems to learn from data and make decisions without explicit programming. It uses algorithms that recognize patterns within large datasets and applies those patterns to new data inputs.
This capability is especially critical in the context of AI-driven analytics, where the volume of data generated daily can be overwhelming. Machine learning algorithms efficiently categorize, cluster, and analyze data, leading to faster insights and better outcomes.
By embracing machine learning, organizations can shift from reactive to proactive modes of operation. Rather than waiting for trends to emerge, businesses can analyze data streams continuously to anticipate and respond to changes in the marketplace.
Enhancing Data Processing and Analysis
One of the most significant contributions of machine learning to AI-driven analytics is its ability to enhance data processing and analysis.
Through supervised and unsupervised learning techniques, machine learning algorithms can sift through enormous datasets with speed and precision. Supervised learning involves training the model on labeled data, while unsupervised learning enables the model to identify patterns without prior labels.
For example, in customer segmentation, machine learning can analyze demographic and behavioral data to group customers effectively. As the model learns from new data, it refines its segmentations, allowing marketers to target consumers more effectively.
Additionally, machine learning algorithms can adapt to evolving market conditions. When new data becomes available, these models adjust their predictions, ensuring organizations always have access to the latest insights relevant to their needs.
Predictive Capabilities and Decision-Making
The predictive capabilities of machine learning are among its most valuable assets in AI-driven analytics. Businesses can use these capabilities to forecast future events based on historical data patterns.
For instance, in the energy sector, companies can leverage machine learning models to predict energy consumption levels based on weather forecasts and historical usage data. Accurate predictions enable these firms to allocate resources efficiently, preventing wastage and maximizing profitability.
Furthermore, machine learning supports decision-making processes by presenting recommendations derived from data analysis. These recommendations can range from operational adjustments to marketing strategies, all tailored based on thorough data assessments.
Ultimately, the synergy between machine learning and AI-driven analytics cultivates an environment where data-driven decision-making becomes the norm, leading to smarter business practices and enhanced organizational performance.
Implementing AI-driven Analytics Strategies
Adopting AI-driven analytics requires a well-thought-out strategy that aligns with an organization’s goals. This section outlines the key steps needed to implement effective AI-driven analytics strategies.
Building a Data-Driven Culture
The first step towards successful implementation is fostering a data-driven culture within the organization.
Leadership must advocate for data analytics as a cornerstone of business strategy. This ethos should be instilled across all levels of the organization, encouraging employees to prioritize data in their decision-making processes.
Training sessions and workshops can equip staff with the necessary skills to utilize AI-driven analytics tools effectively. Empowering employees to engage with data fosters a sense of ownership and accountability, which is essential for cultivating a culture that values data insights.
Moreover, recognizing and rewarding data-driven initiatives can reinforce the importance of analytics within the organization. Celebrating successful projects driven by AI insights motivates teams to continue exploring the potential of data in their work.
Selecting the Right Tools and Technologies
Choosing the right tools and technologies is crucial for successful AI-driven analytics implementation.
Organizations should evaluate their existing infrastructure to determine whether it supports advanced analytics solutions. Cloud-based platforms offer scalability, making them ideal for managing large datasets and complex analytics processes.
Selecting user-friendly tools that integrate seamlessly with existing workflows is essential to encourage employee adoption. Comprehensive software solutions tailored to various analytical needs can streamline processes and enhance productivity.
Additionally, organizations must consider data security and compliance when selecting analytics tools. Ensuring that chosen platforms adhere to privacy regulations protects both the organization and its customers.
Developing a Clear Analytical Framework
Having a clear analytical framework is paramount for directing AI-driven analytics efforts effectively.
Organizations should define their objectives for using AI-driven analytics. Whether the goal is improving customer engagement, enhancing operational efficiency, or increasing sales, clarity ensures that all analytical endeavors align with the overarching business strategy.
Moreover, establishing key performance indicators (KPIs) allows organizations to measure the effectiveness of their analytics initiatives. Regularly assessing progress against these KPIs facilitates adjustments to tactics and strategies based on performance insights.
Finally, continuous evaluation and refinement of the analytical framework ensure that the organization remains responsive to changing market conditions and internal needs. The iterative nature of this process nurtures sustained growth and innovation.
Overcoming Challenges in AI-driven Analytics Adoption
While the benefits of AI-driven analytics are profound, organizations may encounter challenges during adoption. This section addresses common hurdles and offers strategies to overcome them.
Data Quality and Accessibility
One of the primary challenges faced by organizations is ensuring the quality and accessibility of data.
Data silos often inhibit effective analysis. When data is fragmented across different departments or systems, comprehensive analytics becomes challenging. To combat this issue, organizations should invest in centralized data management systems that promote data sharing and collaboration.
Moreover, maintaining high data quality requires ongoing efforts. Organizations should establish rigorous data governance practices to monitor data integrity and accuracy regularly. This includes implementing data validation protocols to identify and rectify inconsistencies promptly.
A commitment to quality data lays the foundation for reliable AI-driven analytics outcomes, ensuring insights derived from analysis are actionable and relevant.
Talent Shortages and Expertise Gaps
Another pressing challenge is the shortage of talent and expertise in AI-driven analytics.
Organizations often struggle to find qualified professionals with the requisite skills in data science, machine learning, and analytics. To address this talent gap, organizations should look to upskill existing employees through training programs and partnerships with educational institutions.
Additionally, fostering a collaborative environment encourages knowledge-sharing among team members. Creating cross-functional teams with diverse skill sets enriches analytical capabilities and drives innovation.
Investing in talent development not only fills immediate skill gaps but also establishes a pipeline of knowledgeable employees who can guide future AI-driven analytics initiatives.
Resistance to Change
Resistance to change can hinder the successful adoption of AI-driven analytics within organizations.
Employees accustomed to traditional decision-making methods may be hesitant to embrace new technologies and processes. Effective change management strategies are vital for easing these transitions.
Communicating the benefits of AI-driven analytics clearly and transparently can alleviate concerns. Highlighting success stories that showcase positive outcomes resulting from data-driven decisions fosters enthusiasm for adopting new approaches.
Moreover, involving employees in the implementation process encourages buy-in. Providing opportunities for feedback and input creates a sense of ownership over the transition, making it easier for teams to adapt to new analytics methodologies.
Conclusion
As organizations navigate an increasingly complex landscape, AI-driven analytics emerges as a powerful tool for unlocking insights and driving informed decision-making. By integrating advanced technologies such as machine learning, companies can harness vast amounts of data to identify trends, predict future outcomes, and optimize operations.