12 Myths of Data analytics Debunked
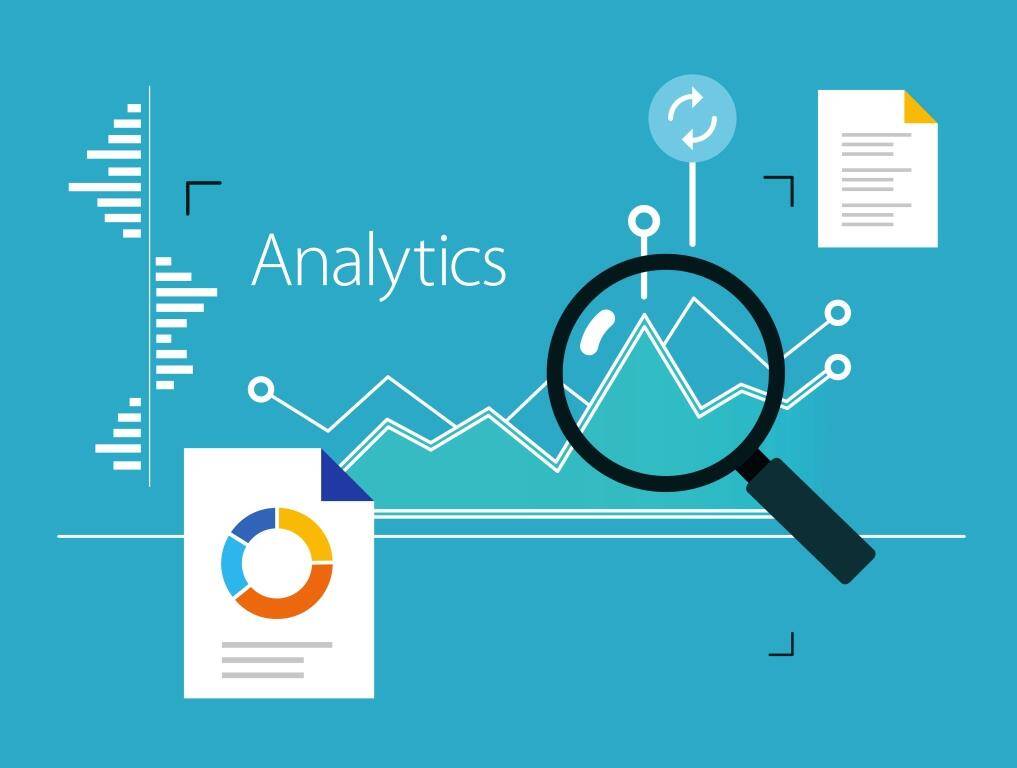
In IT, the bigger the hype, the greater the misconceptions, and data analytics is no exception. Here’s a no-bull look at how to leverage data science to deliver bona fide business results.
Data analytics, one of the hottest facets of information technology today, can result in significant business gains, but misperceptions can get in the way of a smooth and timely delivery of analytical capabilities that might benefit business users and ultimately customers.
As organizations create or expand their analytics strategies, here are a dozen myths they might want to keep in mind.
Myth 1: Data Analytics Requires a Major Investment
These days it seems as if every technology endeavor must pass through a filter of financial soundness. “How much will it cost?” is one of the first questions IT and business managers get when they propose launching a project or deploying a new tool.
Some assume that data analytics is by nature a costly undertaking and therefore limited to organizations with big budgets or lots of internal resources. But not all data analytics efforts require a major investment, says Deep Varma, vice president of engineering at Trulia, a provider of mobile and online real estate services.
“Nowadays there are so many open source as well as other tools available in the marketplace that can help you start to show the value of data analytics,” Varma says. “You need to have a good understanding of your internal data storage and what problem you’re trying to solve. Cloud is also making it easy to try analytics to solve a business problem.
Modern analytics “are based on cloud systems and big data architecture, which by definition are quite less expensive than traditional data warehouse systems,” adds Beatriz Sanz Saiz, global leader of analytics at advisory firm EY.
“Also, data and analytics are typically applied to achieve three outcomes: improve process efficiency, revenue growth, and proactive risk management,” Saiz says. “So overall, the application of data and analytics drive significant [cost] benefits to any company.”
Myth 2: You Need Big data to Perform Analytics
For many, the concepts of big data and analytics go hand in hand. The thinking is that organizations need to gather enormous volumes of data before performing analytics in order to generate business insights, improve decision making, etc.
Certainly the benefits of big data analytics have been well established, and companies with the resources can indeed gain significant competitive advantage by leveraging their data stores as part of analytics efforts. But the idea that big data is a must for analytics is not true.
“Oftentimes people try to capture as much data as they can get; they hear ‘big data and get excited,” says Tim Johnson, executive director of business intelligence at staffing company Allegis Global Solutions. “The misconception is that the more data the better, and that machines will sort it all out.”
However, instead of more data, analysts need specific data. “Ninety-five percent of users are looking for information that’s relevant to their jobs and supports decision making and improved performance,” Johnson says. Instead of focusing on more data, organizations must consider the business users to determine not just what data they need to access, but how it’s presented.
“Providing access to every bit of information and in multiple formats can be overwhelming and actually stifle adoption,” Johnson says. “Instead, find out what’s important to them and how you can present that information to them in the simplest possible format.”
Myth 3: Analytics Removes Human Bias
The way automated systems perform is not supposed to be biased. But technology is built by humans, so eliminating all bias is nearly impossible. Some think analytics and machine learning remove human bias.
“Unfortunately, this is not true at all,” says Mike Mason, head of technology at ThoughtWorks, a global technology consultancy. “Algorithms and analytics are tuned using ‘training data’ and will reproduce whatever characteristics that training data has.”
In some cases, this will introduce a benign bias into analytics results; in other cases, a more severe bias, Mason says. “Just because ‘the algorithm said so’ doesn’t mean the answer is fair or useful,” he says.
Read more: The Ultimate Tech Stack for Real-Time Analytics
Myth 4: The Best Algorithm Always Wins
It actually turns out that with enough data, “sometimes the algorithm doesn’t matter,” Mason says. In an IEEE article he cites, “The Unreasonable Effectiveness of Data,” Google engineers argue that simple statistical models, coupled with extremely large amounts of data, achieve better results than an “intellectually superior” model containing lots of features and summarizations.
“In some cases, just crunching a bigger pile of data will achieve the best results,” Mason says.
Myth 5: Algorithms are Fail Safe
People inherent trust statistical models and algorithms to a high degree, and as organizations build their analytics programs they increasingly rely on sophisticated models to support decision making, Johnson says.
“Because people don’t understand the models, algorithms, and other advanced data science practices, they place their trust in them,” Johnson says. Users don’t feel like they have the knowledge to challenge the models, so instead they must trust the “smart people” who built them, he says.
“For the last 50 to 60 years we’ve heard AI will take over in 20 years, and we’ll continue to hear people say that,” Johnson says. “There is still a lot of ground to cover before we can blatantly trust machine learning and the results. Until then, we need to challenge the people who build algorithms and models to explain how answers are reached. It’s not that we can’t depend on the results; it’s that we need transparency so that we can trust and verify analytics.”
Myth 6: Data science is a mysterious ‘black art’
The discipline of data science has received lots of attention in recent years and sometimes generates confusion as to exactly what it is. Basically, it involves the use of algorithms to find patterns in data.
“Data science seems mysterious because these algorithms are capable of analyzing more variables and larger data sets than the human mind can comprehend,” says Trevor Schulze, CIO at data storage company Micron.
“As computing power and memory have scaled in recent years, we are now able to quickly solve problems that would have been impractical to solve with any technique just 10 years ago,” Schulze says. “Data science is the natural evolution of statistical inference techniques that have been well understood for decades. There is no mystery to data science, once you understand the math.”
Read more: Top 20 Most Popular Data Science Tools for 2024
Myth 7: To do more data science, you need more data scientists
Data scientists are among the most in-demand of all technology professionals these days. Perhaps organizations can get by with fewer of these professionals if they redirect what they’re working on.
“A lot of data scientist time is spent on non-value-add activities like finding data sets, getting the data to a place where it can be worked on, and transforming and cleaning it,” Mason says. “Given how hard it is to hire data scientists, those low-value tasks aren’t what you want them working on.
“Uber’s Michelangelo platform allows data scientists to focus on feature engineering, extraction, and analysis, rather than schlepping data around, and so allows them to be massively more productive,” Mason says.
Myth 8: Analytics takes too long
These days getting things done quickly — whether it’s rushing a product or service to market or responding to a customer inquiry in near real time — is a big competitive consideration for companies. Analytics sounds like something that takes a long time to perform, running counter to the goal of achieving speed and agility.
“The myth still exists that these types of projects take too long and are quite complex,” Saiz says. “At the end of the day it’s all about talent. With the right mix of skills and the application of agile methodologies, big questions can be answered in days or weeks, not months.”
Myth 9: Technology is the hard part
With the ever-increasing number of technologies available today, selecting the right combination of tools to deploy and integrate to get the desired results from the analytics team is no walk in the park, says James Burke, director of IT sourcing and digital advisory services at consulting firm ISG.
The real hard part, however, is “putting together the organizational structure and operating model to put all of what is required from a people, process, technology perspective together,” Burke says. “Also, how you do this inside of or ‘next to’ your existing organization is what seems to be most difficult for organizations.”
It’s also important to not assume that analytics tools will do all the work. “Technology alone never solves any business problem,” says Greg Layok, senior director of consulting firm West Monroe’s technology practice. “In the rush to create data lakes, organizations are instead ending up with swamps, or a morass of information that is too difficult for anyone to figure out.”
Technology doesn’t solve analytics problems, Layok says. “First, identify a business problem, then ask, ‘What data do I need to solve this?” he says. “This will help you identify the gaps of data within your organization.”
Myth 10: Data analytics should be a separate department
In some organizations data analytics operates as a department on its own, and in others it is deeply embedded into a cross-functional team, says Steven Mast, president and chief innovation officer at Delvinia, a consulting and data collection company.
“However, with the explosion of data across all areas of business and the speed at which change is occurring, the department model does not work,” Mast says. “As organizations become more customer-centric, data-driven analytics specialists should be at the core of a business unit, not operating as a department that you call for support.”
Many of the complex problems organizations face today are within the business units, and many solutions to these problems are hidden in data, Mast says. “Data scientists and specialists, working closely with these business units, and using large data sets and artificial intelligence, will be key to incubating the next generation of products, services, and customer experiences.”
Myth 11: Analytics is just for PhDs
It’s great to have lots of well-educated people on the analytics team, but it’s not a requirement for success.
“Companies tend to think that without PhDs on board they won’t be able to perform best-in-class analytics,” Saiz says. “Modern analytics demand a blend of skills — those who are savvy in emerging technologies and open source software. Building ‘pods’ with different skills [including] big data architects, data engineers, data scientists, experts in data visualization, and more, is what makes the difference.”
Myth 12: AI will destroy jobs and wreck the economy
The introduction of new technology historically has disrupted many jobs and industries, and there are fears that artificial intellgience will eliminate the need for people to perform certain tasks.
“Artificial intelligence solutions are much better than people at solving certain kinds of problems,” Schulze says. “AI can read faster, remember more, and calculate complex mathematical relationships better than any person. However, AI is not capable of dealing with truly novel situations, which is where humans excel.”
To be sure, Schulze says, certain jobs have already been lost or diminished by the growth of AI, and others will follow. “Still, our human capacity to understand and work around completely unforeseen circumstances will not be replaced by any currently understood AI technology,” he says. “For the foreseeable future, the most effective approach to AI will be to augment human abilities with AI systems that perform some of the ‘heavy lifting’ where algorithms outperform people. Though many jobs will change as a result of AI, people will continue to be a critical part of this business ecosystem.”