Transforming Healthcare with AI in Medical Imaging
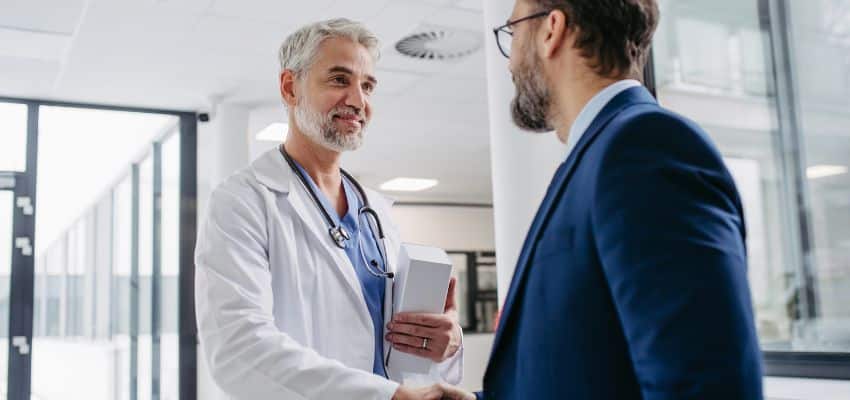
The integration of AI in Medical Imaging represents a paradigm shift in the healthcare landscape. As medical imaging continues to evolve, artificial intelligence (AI) offers unprecedented opportunities to enhance diagnostic accuracy, streamline workflows, and ultimately improve patient outcomes. This article delves into the transformative effects of AI in medical imaging, exploring its applications, benefits, challenges, and future potential.
The Role of AI in Enhancing Diagnostic Accuracy
As healthcare technology advances, so too does the complexity of tasks involved in diagnosing diseases through imaging.
Revolutionizing Image Analysis
AI algorithms are adept at analyzing images with speed and precision that often surpasses human capabilities.
Moreover, AI-powered image analysis can assist radiologists by providing second opinions, which is particularly beneficial in complex cases. By flagging potential issues, AI acts as a safety net, ensuring that no critical findings slip through unnoticed. This collaborative approach enhances diagnostic confidence among clinicians while optimizing workflow efficiency.
Reducing Human Error
Human error remains a significant concern within medical imaging. Fatigue, distraction, and variability in expertise can all contribute to misdiagnoses.
Additionally, AI can help prioritize cases based on severity or complexity, allowing radiologists to focus their attention where it is most needed. By easing the cognitive load on healthcare professionals, AI contributes to more accurate assessments and optimal treatment plans for patients.
Improving Early Detection Rates
One of the most compelling advantages of AI in medical imaging is its capacity for early detection of diseases.
This proactive approach not only supports better health outcomes but also fosters a culture of preventive care within medicine. By leveraging AI technologies, healthcare providers can move toward a model that prioritizes early intervention, ultimately saving lives and reducing the burden of advanced disease.
Streamlining Workflow and Efficiency in Radiology
As the demand for medical imaging services grows, the pressure on radiology departments intensifies.
Automating Routine Tasks
AI’s ability to automate routine tasks is one of its most significant contributions to enhancing operational efficiency.
Furthermore, AI can assist with preliminary report generation by automatically analyzing images and summarizing findings. This not only saves time for radiologists but also allows for quicker communication of results to referring physicians and patients. In this way, AI can transform the radiology department into a more agile and responsive unit capable of meeting the demands of modern healthcare.
Enhancing Interoperability
AI can facilitate seamless communication between disparate imaging modalities, electronic health records, and clinical decision support systems. By ensuring that data flows smoothly across different systems, AI contributes to a more integrated healthcare experience.
Optimizing Resource Allocation
Efficient resource allocation is crucial for healthcare institutions to maximize their impact.
AI can analyze historical data to identify trends and patterns in imaging utilization, enabling administrators to make informed decisions about resource distribution. Whether it’s determining when to expand imaging services or assessing the need for new equipment, AI’s insights provide a roadmap for strategic planning.
By aligning resources with patient needs, healthcare facilities can ensure they are adequately prepared to provide timely care. This not only improves the overall patient experience but also bolsters institutional reputation and financial viability.
Ethical Considerations and Challenges in AI Implementation
While the prospects for AI in Medical Imaging are promising, ethical considerations surrounding its implementation cannot be overlooked.
Data Privacy and Security
The integration of AI necessitates the collection and analysis of vast amounts of sensitive patient data.
Ensuring the privacy and security of this information is paramount. Healthcare organizations must adhere to stringent regulations, such as HIPAA in the United States, to protect patient confidentiality. Moreover, AI systems must be designed with robust security measures to prevent unauthorized access or data breaches.
Transparency around how data is used and stored is essential in building trust with patients. Institutions that effectively communicate their commitment to data protection will foster greater acceptance of AI technologies within the healthcare community.
Bias and Fairness in AI Algorithms
AI systems are only as good as the data they are trained on.
If training datasets are not representative of the diverse patient populations they serve, AI algorithms may inadvertently perpetuate biases. This could lead to disparities in care and adversely affect certain demographic groups. It is vital for developers to ensure that training datasets encompass a wide range of demographics so that AI models can provide equitable outcomes.
Regular audits and assessments of AI systems should be conducted to identify and rectify any biases that may emerge over time. By committing to fairness and inclusivity, healthcare organizations can leverage AI responsibly and ethically.
The Role of Physicians in AI Decision-Making
As AI systems become more integrated into clinical workflows, questions arise regarding the role of healthcare professionals in the decision-making process.
While AI can offer valuable insights, it should not replace the expertise and judgment of physicians. Instead, AI should serve as an augmentative tool, empowering clinicians to make informed decisions rather than dictating actions.
It is important for healthcare providers to maintain a sense of agency in the face of AI advancements. Proper training and education on using AI tools will equip healthcare professionals to interpret AI-generated recommendations critically and integrate them meaningfully into their practice.
The Future of AI in Medical Imaging
Looking ahead, the future of AI in medical imaging holds immense potential for innovation and improvement.
Advancements in Deep Learning Techniques
Continuous advancements in deep learning techniques are expected to refine the capabilities of AI in medical imaging further.
Researchers are exploring novel architectures and training methodologies that can enhance the performance of AI models. These advancements will enable AI systems to handle increasingly complex imaging tasks, expanding their applicability across various medical specialties.
As AI continues to evolve, it will likely adopt a more personalized approach, tailoring analyses to individual patient profiles and specific clinical contexts. This shift toward precision medicine will usher in a new era of targeted diagnostics and interventions.
Integration with Other Technologies
The intersection of AI with other emerging technologies presents exciting possibilities for medical imaging.
For example, combining AI with augmented reality (AR) and virtual reality (VR) can revolutionize surgical planning and educational training. Surgeons could use AR overlays to visualize critical structures during procedures, improving accuracy and reducing complications.
Similarly, integrating AI with wearable devices and telemedicine platforms can enhance remote imaging capabilities, enabling healthcare providers to monitor patients and diagnose conditions from afar. These synergies between AI and complementary technologies have the potential to transform patient care delivery.
Global Accessibility and Equity
AI in medical imaging also has the power to address global health disparities.
By improving the efficiency and accuracy of diagnoses, AI can empower low-resource healthcare settings to deliver high-quality imaging services. Additionally, AI systems can be deployed in telehealth applications, facilitating access to expert consultations for underserved populations.
Efforts to democratize AI technologies in healthcare should focus on addressing infrastructural challenges and ensuring that marginalized communities benefit from these advancements. A commitment to equity in healthcare will shape the future of AI in medical imaging, fostering a more inclusive system for all patients.
Conclusion
The integration of AI in Medical Imaging is reshaping the healthcare landscape in profound ways. From enhancing diagnostic accuracy to streamlining workflows, the potential benefits of AI are vast and varied. However, ethical considerations, bias mitigation, and the preservation of the physician’s role remain critical challenges that must be addressed as we navigate this technological evolution.